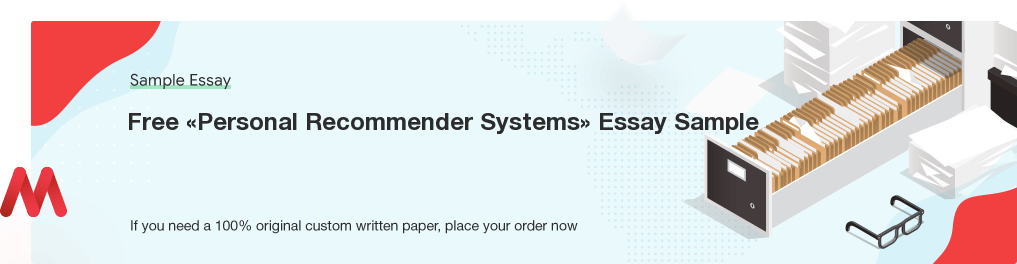
Custom «Personal Recommender Systems» Essay Paper
Personalized recommender systems have significantly transformed the way online users find information, products, or even people. In fact, these systems are artificial intelligence (AI) systems that study the behavioral patterns of online users in order to identify and suggest what a person may appreciate from a collection of items that has never been purchased or experienced by him or her. These systems employ prediction algorithms and data mining techniques, to predict the interest or preferences of users on products, services, and information from a wide variety. The exponential growth of information on the Internet, as well as the increasing number of visitors to e-commerce and m-commerce platforms, presents some key challenges to both the users and personal recommender systems. Some of the challenges include coping with numerous participants, attributes, and domains, handling many recommendations, and producing accurate recommendations even if the data are inadequate. Central to these challenges is the growing demand for social media and social networking sites. For this reason, innovative recommender systems or technologies are required to produce an excellent recommendation for various data sets.
The research is based on the studies conducted by various scholars and industry reports. Previous research has shed a little light on the benefits and challenges of the recommender systems in the field of m-commerce. To that end, this research focuses on the technology in the realm of mobile commerce. The paper provides an overview of the personal recommender systems and discusses the present generation of recommendation approaches that are characteristically classified into collaborative, content-based, and hybrid recommendation methods. It focuses on the collaborative filtering approach including its operation, usage, and evaluation, as well as its strengths and weaknesses. Various resources including journal articles and other scholarly resources related to the field of recommender systems are used.
Literature Review
Electronic commerce is an approach to conducting business over the Internet using computerized devices (Laudon & Traver, 2007). M-commerce is a variant of electronic commerce that uses wireless networks and mobile devices. Examples of commerce over mobile networks and devices include mobile banking, news reading, and location-based services. M-commerce uses recommender systems, which are designed for mobile devices. According to Ricci, Rokach, and Shapira (2011), recommender systems (RS) are types of the artificial intelligence (AI) software tools, which are used to provide suggestions concerning the items to be interesting to particular users. In their simplest structure, personalized recommender systems offer a recommendation in the form of ranked lists of products or items (Aldrich, 2011; Burke, Felfernig, & Göker, 2011). In this context and henceforth, the term item refers to anything recommended by the system to users. Recommender systems analyze users’ online behavior and demographic information and automatically create personalized or customized recommendations for the most appropriate product and services (Jannach, Zanker, & Friedrich, 2010). Along with the common items such as movies and books, the recommender technology is projected to play a growing role in e-commerce and m-commerce as more new applications emerge (Ricci, Rokach, & Shapira, 2011). For instance, people are expected to employ recommender systems to search for the jobs or suppliers.
Limited Time offer!
Get 19% OFF
The central technologies behind these systems have evolved in the last two decades into a rich set of software tools and techniques that enable researchers and practitioners to develop efficient recommenders. As noted by Burke, Felfernig, and Göker (2011), the field of recommender system was introduced in 1995 and, since then, had grown rapidly to address various problems using different algorithms. Research in the field has incorporated a range of AI techniques such as data mining, machine learning, constraint satisfaction, case-based reasoning, and user modeling, among others. Burke, Felfernig, and Göker (2011) pointed out that personalized recommendation systems are an integral part of various online e-commerce applications. In this context, they help entities reduce the time spent by the end-users while looking for items. For instance, Amazon provides its online customers with a list of products linked to their personal recommender systems. The end users rate products based on their interests or preferences. In turn, new users can be given recommendations, according to the similar behavioral patterns. Amazon’s recommendation system is based on a number of constructs: items bought by a user in the past, items in a user’s virtual shopping cart, items viewed or purchased by other customers, and the items that the same users have rated or liked (Mangalindan, 2012). At root, the system employs the item-to-item collaborative filtering approach. The approach is used to customize the browsing experience for customers that revisit the website. From Amazon’s success in the online retailer industry, one can infer that the recommendation system is effective. A lot of Amazon’s growth is attributed to the way the company has integrated its recommender systems into virtually all parts of its purchasing process.
We Provide 24/7 Support
Have you got any questions?
Typically, the recommender solutions focus on specific items, such as news, books, and movies. In the same vein, the design, graphical user interface (GUI), and the technique used to generate recommendations are customized in order to ensure that effective or valuable suggestions are provided for each type of product. However, it should be noted that designing and developing a mobile recommender system is a very demanding process because it calls for knowledge and skills beyond the fields of computer science. Additionally, the algorithms used in the conventional computing environment cannot be directly transferred to mobile devices. The transfer constraints are linked to the fact that mobile devices have different characteristics, needs, and specific limitations. Therefore, recommender systems employ numerous algorithms. They include collaborative filtering (user-user and item-item), content-based filtering, and dimensionality reduction, among others (Ghazanfar, Prügel-Bennett, & Szedmak, 2012; Lü et al., 2012). Recommender systems use various algorithms to collect the user data and generate recommendations. There are three most popular recommendation systems algorithms, but this paper focuses on the collaborative filtering one.
Benefit from Our Service: Save 25%
Along with the first order offer - 15% discount, you save extra 10% since we provide 300 words/page instead of 275 words/page
Collaborative Filtering (CF)
Collaborative filtering (CF) is one of the popular design approaches based on clustering and analyzing the information, according to the users preferences, behaviors, and interests. The clustered data are used in predicting what new users are likely to consider valuable based on the similarities in the preferences or interests of the previous customers (Ghazanfar, Prügel-Bennett, & Szedmak, 2012). The basic concept of the CF systems is to find a group of users that share similar interests with a past user. The rationale of these systems is an assumption that users with similar tastes or preferences in the past are likely to have the same preferences in the future. Pure CF systems use the user-input matrix with the user rating as the primary input and generate a recommendation based on similarities to the past users (Konstan & Riedl, 2012; Ricci, Rokach, & Shapira, 2011). These similarity methods include cosine and modified cosine similarity (Jannach, Zanker, & Friedrich, 2010).
The central advantage of this method is that it does not rely entirely on the analysis power of machines; hence, it is capable of generating a recommendation on different items such as movies without the need to learn about them. Collaborative filtering employs the Pearson correlation and the k-nearest neighbor approaches on the assumption that similar preferences or interests in the past are much likely to agree in the future (Dehuri, 2012). As of consequence, they are likely to choose or prefer similar types of items as they used to do in the past. PRS compares the information collected to both comparable and disparate information clustered from other users and computes a list of recommended items for the new user. Amazon employs an item-to-item comparative filtering approach (Ricci, Rokach, & Shapira, 2011). In turn, social networking sites (SNSs), including Facebook and MySpace, employ collaborative filtering approach to recommend new friends. Recommender systems have the capability of archiving the user data, simulating and modeling the stored information, and reporting for customized results. Such companies as Google, Netflix, Amazon, and Pandora employ personal recommender systems in order to enhance customer’s satisfaction and improve their sales (Burke, Felfernig, & Göker, 2011; Konstan & Riedl, 2012). Besides improving customers’ satisfaction, PRSs are used beyond m-commerce and e-commerce environments for assisting investors in choosing investments or stocks. As noted by Burke, Felfernig, and Göker (2011), companies use PRSs to collect valuable user data that can be employed in the business intelligence (BI) systems to offer certain services or products to consumers.
VIP Services
Get
extended REVISION
Get
SMS NOTIFICATIONS
Get an order
Proofread by editor
Get an order prepared
by Top 30 writers
Get a full
PDF plagiarism report
Get
VIP Support
VIP SERVICES
PACKAGE 23.82 USD
Trends and Characteristics of the Current Recommender Solutions
It is evident that e-commerce and m-commerce have some similarities between services and operational perspectives. However, mobile commerce has specific technology motivated business opportunities with unique opportunities, characteristics, functions, and challenges. In mobile commerce, the needs location is often found in mobile finance and tourism services. The term characteristics refer to the processor, user interface, and memory capabilities. In this context, limitations refer to the boundaries found in GPS, GSM, and Wi-Fi systems (Polatidis & Georgiadis, 2013). Despite this fact, the provided benefits are important, including ubiquity and location-based services. The two factors form the pillar of the mobile recommender systems. Personalized recommender services are enabled by recommender systems; thus, they serve as an illustration of a successful application of the recommender technology in e-commerce. In the m-commerce realm, PRS are still only emerging. Nevertheless, personalization, user modeling, and adaptation techniques have gained popularity in the mainstream. A sudden increase in SNSs has generated an enormous amount of data (Jannach, Zanker, & Friedrich, 2010; Polatidis & Georgiadis, 2013). Data inflation has also been stimulated by a remarkable growth in the computational power of the mobile networks and handheld devices. In the same context, there is an increase in the desire of users to personalize their news, e-commerce, and m-commerce sites (Zenebe, Ozok, & Norcio, 2005). The prospective value of personalization has become apparent both as a product for the enjoyment and benefit of users, as well as enablers of innovative or high-quality service. From the business perspective, personalization serves as a strategic opportunity for online commercial entities to expand and enhance businesses.
Top 30
writers
Get the most experienced writer
in the relevant discipline!
An interesting aspect of personal recommender systems is that they attract the interest of companies and industry while posting exciting scientific and research challenges. Despite recording a significant progress in the PRS research community, as well as collective efforts to realize the benefits of new recommended solutions, there are still imperative gaps that make personalization and adjustment difficult for the end-users. Most research efforts tend to focus on the narrow problems, including applicative issues and improvement of the accuracy of the existing recommenders (Aldrich, 2011; Jannach, Zanker, & Friedrich, 2010). This fact constrains the range of the other application areas, in which recommender systems can be used. For this reason, scholars and practitioners should take an informed perspective on the research conducted in a recommender system. The research focuses on academic papers and journals with compelling concepts that stimulate discussion in the field. According to Aldrich (2011), the commercially available recommender systems are similar in composition. Firstly, the commercialized solutions have proprietary recommender engines. Moreover, the user data collected by them are usually hosted by the solution vendor. It follows that most modern recommendation systems exploit server virtualization and cloud computing technologies. Commercial solutions also have services that process bulk user data such as order history, as well as request and return recommendations. Further, vendors provide the client care service including teaching and guiding their users on the optimum use of the supplier’s recommendation engine. Most importantly, they integrate some reporting tools with the capability of exporting the user data into various standardized formats for compatibility, such as the comma-separated value files (CSV) (Aldrich, 2011).
VIP Support
VIP support services:
extra attention is guaranteed!
As of this writing, e-commerce and m-commerce sites had the ability to collect and use the end-users location information in order to provide informed recommendations. Recommenders and ubiquitous systems can collect and store users’ data based on their geographical locations (Mettouris & Papadopoulos, 2014). Such information can be used to complement recommendations in the real time. In this context, complex filtering techniques and algorithms are used to provide suggestions based on the user's previous input and location. Some of the challenges facing this approach are privacy concerns, device power management, and limitations of the wireless connectivity, among other issues (Mettouris & Papadopoulos, 2014).
Mobile Environment
The use of mobile devices in line with the rapid advancement in the Internet-based technologies has also contributed to the information overload problem (Aldrich, 2011). Personalized recommender systems assist mobile users in choosing relevant data from a pool of information. In the mobile realm, there are various factors that are crucial to information retrieval, including screen size, power management, processor speed, memory, and location (Polatidis & Georgiadis, 2013). The need for m-commerce has stimulated the use of recommender systems and increased the implementation of personalization techniques. Furthermore, their application has been motivated by the advancement in the last mile connectivity, as well as rapid development of mobile technology platforms such as Apple, iPhone, and Google Android (Polatidis & Georgiadis, 2013). The use of the Internet-enabled applications is moving towards the handheld devices. Although such devices come in different forms, this paper narrows them down to mobile phones with the Internet connectivity and features that enable users to access information from the e-commerce sites.
Still have any questions?
,
The Internet-enabled handheld devices have become popular; today, they are considered as the primary platform for the online information assessment (Zenebe, Ozok, & Norcio, 2005; Konstan & Riedl, 2012). However, as the number of the Internet users increases and the information on the Web continues to expand, there is a pressing need to address the challenges that are related to the mobile computing environment. Central to the challenges is the need to tackle information overload alongside associated limitations that most mobile devices have. In response, entities are forced to exploit recommendation technologies (Bassis, Esposito, & Morabito, 2014; Dehuri, 2012). Similarly to the computer world, recommender systems in the mobile environment are concerned with the customization of data about user preferences or interests collected over the Internet. The scope of recommendation is designed to assist a user to make choices on the news to read, items to buy, or people to follow on the social networking sites.
Benefits of Recommender Systems
The recommender technology is essential for not only users but also service providers. The reasons behind the popularity of RSs among online retailers and service providers include an associated increase in sales, improved loyalty, and greater customer satisfaction. By globalizing their operations, companies become dependent upon online transactions such as online selling on a global scale in order to sustain their advantage. E-commerce and m-commerce will make it possible for the international companies to not only access the volatile globalized market but also maintain their existing customers (Laudon & Traver, 2007; Zenebe, Ozok, & Norcio, 2005). In this respect, the threat of competitors becomes moderate because the companies benefit from a higher market share. Moreover, e-commerce and m-commerce can bring down the cost of marketing while increased sales will improve the profit margin. Companies can also respond to customer relationship management (CRM) through employing a personalization approach or implementing a sales-force automation linked to a recommender system.
Try Our Discounts
Try our service with huge discounts
By using the personal recommender technology, an online entity can benefit from a reduction in time to market, as well as cycle time (Huang, Chung, & Chen, 2004; Laudon & Traver, 2007). By implementing certain aspects of the recommender technology in e-commerce and m-commerce, organizations stand a chance of enhancing their supply networks. An effective e-commerce strategy and recommender system can reduce the supply chain delays and eliminate inefficiencies (Laudon & Traver, 2007). In collaboration with the conventional marketing strategy, e-commerce and recommender systems can significantly increase the market efficiencies. For instance, the function of the market can be expedited or improved. Consequently, the cost sales of transactions and distribution can be lowered.
Recommender systems help users to reduce the time that would have been wasted on searching for goods in the extensive e-commerce sites such as Netflix and Amazon. According to Huang, Chung and Chen (2004), recommender systems enable users search information from the various website within the shortest time possible. As of this writing, recommender technology allows users to select preferred products or items after profiling their habits. Given that there is an enormous pool of data and numerous knowledge sources, various companies are supposed to employ algorithms that suit their needs. Collaborative filtering or hybrid systems can be used by the data scientist to mine data and generate valid information for the users (Ghazanfar, Prügel-Bennett, & Szedmak, 2012). Vendors in the recommender system market can develop a commercially available solution in order to improve the efficiency of the existing recommendation systems. The algorithms used by vendors can induce competition among the e-commerce based companies; in turn, it will translate to the improved customer satisfaction. When companies compete in the personalization of services using PRS, consumers benefit. In other words, firms that produce and improve their algorithms stand a higher chance of providing accurate and timely information to its customers. Recommender systems also enable business entities to avail customized information to clients.
Do you need professionally written papers?
Place your order on our website to get help from qualified experts!